Computational imaging has broad applications to fields such as smartphone photography, autonomous driving and medical imaging. In this post, we review computational imaging in general and then introduce Foqus, a Quantonation I portfolio company whose technology improves nuclear magnetic resonance medical imaging, making it faster, cheaper, and higher resolution.
Introduction
Imaging can be broadly defined as the process of producing a visual representation of a scene by collecting a physical signal (light, sound, etc.) – in a nutshell, taking a picture. retrieving optical information. In more technical contexts, it refers to the image formation process, i.e., how information present in a plane is mapped onto another plane: for example, how stars are imaged onto the retina of an observer using a telescope. Once an image is formed on a new plane it can be recorded, in analog or digital form. Imaging also designates the physical and mathematical modelling of the whole image capture process: how light is manipulated by the system, measured and how the subsequent information is processed.
Computational imaging refers to imaging modalities that involve data processing: at the recording step, information is digitized and processed before an image is produced. Though the distinction is somewhat artificial, it can be contrasted to regular imaging, which involves minimal post-processing of the recorded images.
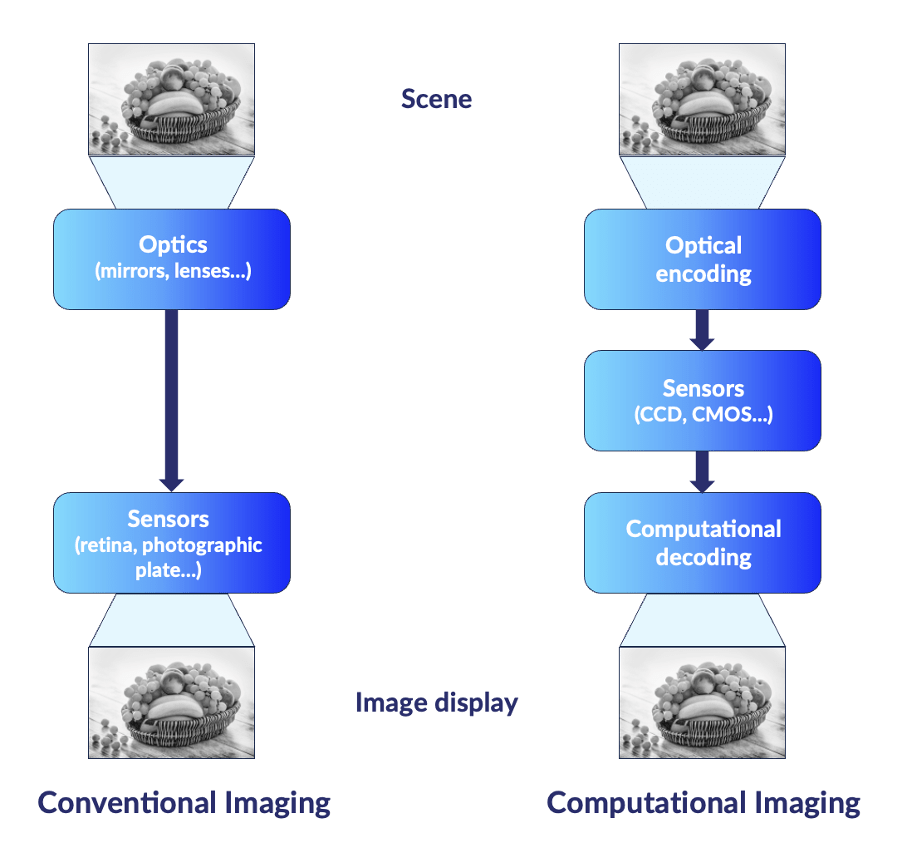
Schematic view of regular and computational imaging systems.
Many computational imaging techniques are already well established. One evidence of this in consumer space is the presence of multiple objectives on the back of most later generation smartphones. The combined signals of these objectives are combined using tailored algorithms to produce pictures of excellent quality. Producing images with comparable metrics (stability – or absence of blurring-, resolution, contrast, etc.) with analog optical components would require far bulkier components: the miniaturization of cameras was made possible by the refinement of the sensors, the multiplication of them and the computational post-processing steps.
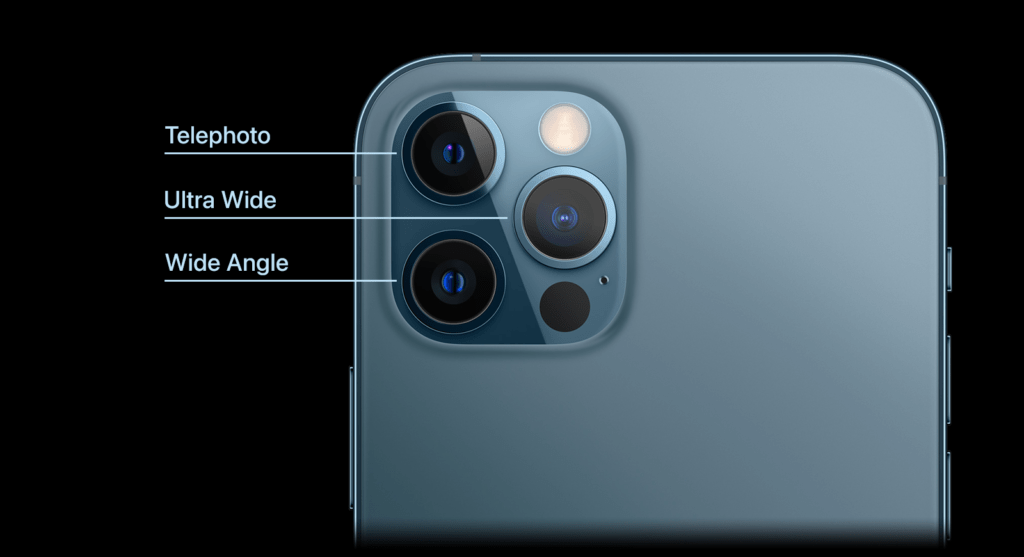
Different objectives on the back of an iPhone 12 Pro Max.
Interdisciplinarity, rather than fundamental physics breakthroughs, are at the heart of computational imaging innovations: applied mathematicians using their signal processing skills on recorded signals, optical engineers twisting the designs of their systems, and computer scientists bringing data processing capabilities never-before envisioned. These new performances have been made possible thanks to the exponential development of computational methods over the last decades. The growing utilization of medical imaging techniques such as Magnetic Resonance Imaging (MRI) and Computed Tomography (CT) scanning is directly linked to the implementation of computational imaging techniques in these fields, allowing them to achieve the performance levels necessary for their adoption in clinical settings. Innovations in computational imaging often make use of a priori information: when one knows beforehand some properties of the signal to be retrieved, the design rules for the imaging and processing setups can be optimized for improved performance.
Advantages brought by computational imaging can reduce the imaging elements’ cost (or ruggedness), increase the acquisition process’s speed, improve images’ resolution, enhance the extraction of the signal from the noise, give access to new wavelength ranges, etc. For instance, these methods have allowed the adoption of the multiple-input multiple-output (MIMO) radar technology, that relies on the use of multiple antennas to transmit and receive simultaneously different waveforms. This allows for enhanced performances: improved image resolutions, extended ranges, better interference immunity [1]. It is widely used in a military context to identify potential targets.
Without being exhaustive, computational imaging techniques can be divided into three comprehensive (and sometimes overlapping) categories. These are by no means standards but provide a useful interpretative framework:
Broad categorization
Algorithm-based
Algorithm-based techniques are nowadays ubiquitous. All digitally acquired images have been algorithmically processed to deliver a better image quality. Once a signal is acquired, data is processed to improve contrast, correct for biases, etc. These algorithm-based techniques, such as deconvolution, are used for aberrations and noise removal [2]. This sub-field is the most mature and versatile one: once data is acquired, it is always possible to post-process it. From a mathematical point of view, whereas some algorithm-based techniques can be as straightforward as filtering, some others use state of the art deep learning techniques [3].
Hardware upgrade:
This category pools the cases where any given part of an imaging system is modified and paired with a corresponding post-processing algorithm. Such improvements require a good understanding of the image formation process in the setup used, as well as the properties of the imaged sample. Structured illumination techniques fit into this category. A very simple illustration (which does not even require post-processing) is oblique illumination, which enhances the contrast and visibility of transparent or colorless objects. Indeed, it allows to reveal refractive index or other optical path differences in specimen which would have appeared transparent in brightfield illumination [4]. More broadly, many structured illumination techniques allow to bypass the diffraction limit of the physical setup used, by shaping the illumination field and algorithmically combining images taken with different illumination patterns [5, 6]. Another direction is the repositioning of hardware – using a given optical hardware in a context different than the one it was originally designed for – to provide for better imaging setups (which can, depending on the context, mean cheaper, faster, more rugged). Examples count wavefront-sensing technologies, originally developed in astronomy to correct for atmospheric aberration and now commercialized across fields as diverse as automotive and microscopy [7], the use of telecommunication optical fibers as antenna arrays [8], or the operation of smartphone objective as microscopes [9, 10].
Co-design approach:
In this third category, the hardware and the processing algorithm are designed jointly. These techniques enable the simultaneous optimization of the optical and computational components of an imaging system, resulting in unprecedented performances and quality levels. In these schemes, the imaging goal and context must be well-specified in advance. One example is super-resolution microscopy: after stimulation with non-trivial light patterns, fluophores present in the imaged sample, with well-characterized emission properties, produce a series of raw images which, after algorithmic processing, reveal a super-resolved picture [11]. Nowadays, low-cost versions of this microscopy technique exist [12,13] and are widely used in biology [14]. Another example is in chemical imaging: often, when one wants to characterize a sample, a rough composition of it is known a priori. Compressive Raman imaging is an emerging technology that exploits a priori information: it is an optical device that takes this type of information into the sampling stage, to perform chemical quantification at the acquisition step, instead of classical post-processing [15,16].
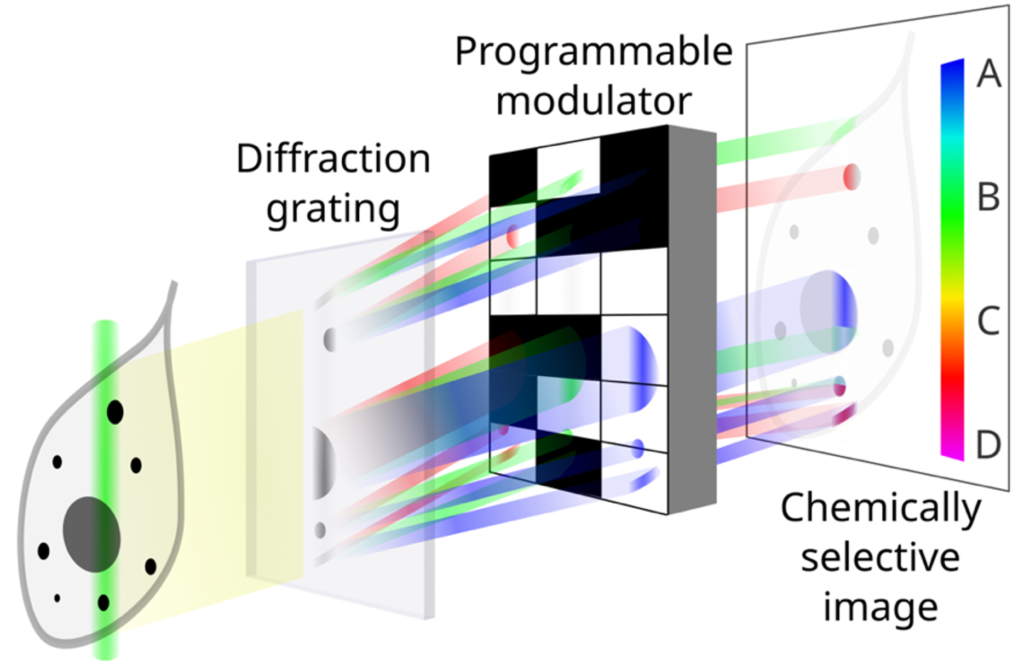
Schematic illustration of the compressive Raman spectrometer [17].
Use cases
Computational imaging systems cover a broad range of applications in various domains such as healthcare, defense, security, entertainment, and education. Here are some examples of the use cases of computational imaging:
Healthcare
The quality and functionality of medical imaging modalities, such as X-ray, CT, MRI, ultrasound or endoscopy can be enhanced by computational imaging techniques. Computational imaging can also help create new modalities, such as digital X-rays, light-sheet microscopy or holographic microscopy. Such innovations help improve diagnoses, treatments monitoring and interventions guidance. The largest companies operating in this field are Thermo Fisher Scientific, GE Healthcare, Philips Healthcare and Siemens Healthineers.
Foqus is a Quantonation I portfolio company whose technology improves nuclear magnetic resonance medical imaging, making it faster, cheaper, and higher resolution.
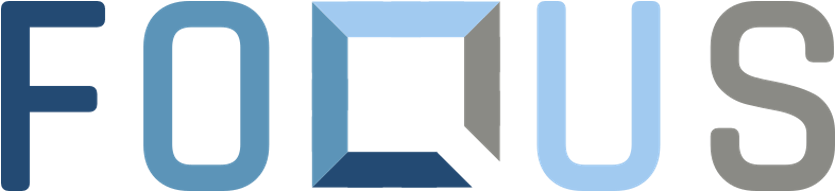
Foqus logo.
Defense
Computational imaging can improve the performance and capabilities of defense systems, such as radar, sonar, lidar, or thermal imaging. The techniques developed in this field have helped with detection and identification of targets to enhance situational awareness, provide stealth and security, and enable remote sensing. Examples include super-resolution and 3D reconstruction of radar signals, or the creation of images from ambient light or sound sources. The main players in this field are defense giants such as Lockheed Martin or start-ups such as Citadel Defense or Metaspectral.
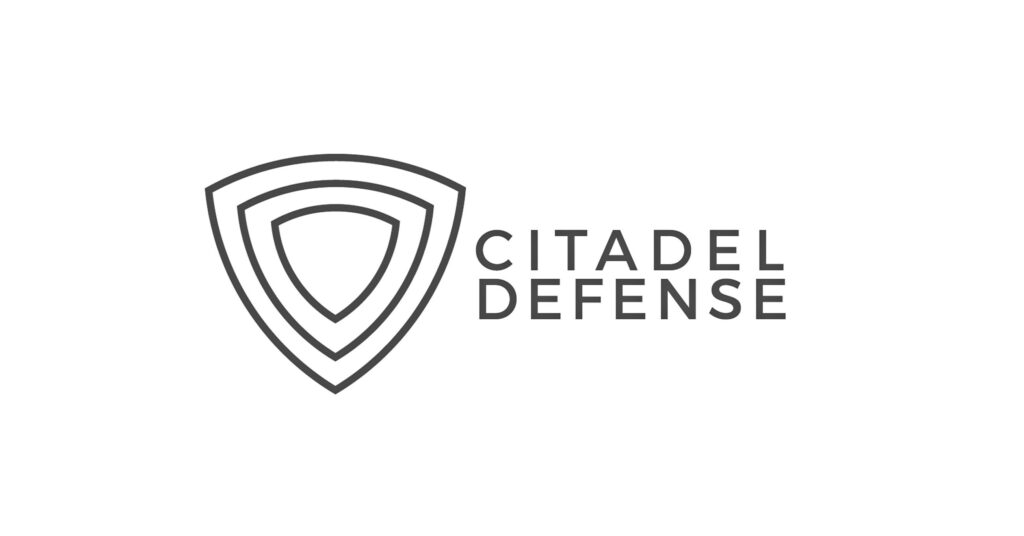
Citadel Defense logo.
Entertainment
The quality and functionality of entertainment devices, such as cameras, smartphones, tablets, TVs and projectors has been improved by computational imaging. It has also enabled the development of new devices, such as virtual reality headsets, holographic displays and more. Computational imaging can contribute to the creation and manipulation of images and videos for artistic purposes, immersive experiences, interactive games and social media. This vertical is dominated by major companies such as technologic and electronic giants (Apple, Google, Microsoft, Facebook, Qualcomm, Nvidia) or imaging ones (Canon, Nikon, Olympus). One late-stage start-up in this field is Light, which develops a multi-lens and multi-sensor camera technology designed for smartphones.
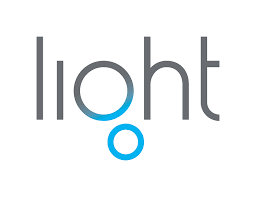
Light logo.
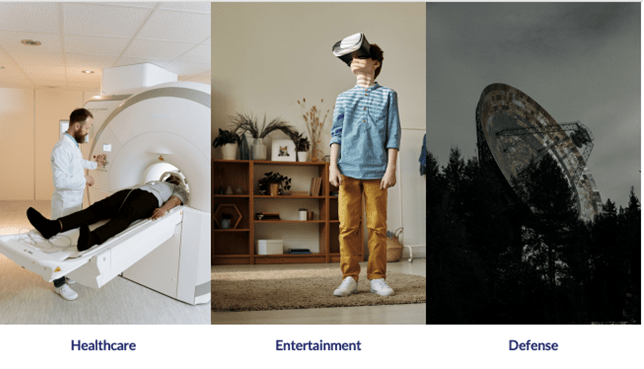
Different use cases of computational imaging: MRI, VR headsets and RADARs.
Drivers
Several drivers can be identified outside those mentioned in our introduction:
Computing power and techniques
Computing capabilities of hardware have drastically improved over the last decades. That fact alone has triggered improvement in computational imaging by supporting more sophisticated data processing techniques: many data processing methods in place today were previously thought to be impractical, or even just impossible, and thus not further researched. Among these data processing ideas, machine learning tools – which are far from limited to imaging – are being actively researched and implemented in the field [18].
Hardware improvement
Almost all the different components involved in imaging setups have witnessed significant improvements. An important example is that of phase modulators (a phase modulator is a device that can modulate the phase profile of a light beam), whose specifications are being constantly improved: different technologies are now available with greater modulation speeds, finer pixel sizes, broader and even new wavelength ranges, finer modulation steps, lower loss levels, multi-mode capabilities [19], etc. Another one is the sensitivity of detectors, which have not only reached the level of single photon detection but are now reaching the photon-number resolving capabilities (e. g. the SPAD sensors crafted by Pi Imaging [20]).
Fabrication processes
Improvements in fabrication processes have also opened new domains: for instance, the surface quality now reached in lenses or mirrors manufacturing supports shaping wavefronts at exquisite levels, removing many aberrations and opening the door to components miniaturization [21]. Deposition layer techniques are reaching the single atom layer and are compatible with a broad range of chemical components [22].
Combinations of techniques
n some systems, information may become disordered or scrambled, but it is not permanently erased and can thus be recovered. The process of retrieval can vary depending on the specific situation, but mathematically, it can be as straightforward as performing a matrix inversion [23].
Most of the new computational imaging systems take advantage of more than one of these elements. Endoscopes, which provide a unique flexible imaging modality, which are used in medicine but also inspection for different industries [24], have been made possible by the great progresses made in fiber fabrication, with near-perfect arbitrary indices profiles, as well as post-sensing data processing [25]. One final trend important to underline is the integration of the calibration and interpretation steps to the imaging process as a whole. For instance, with setups integrating machine learning, the learning step must of course be done before the setup comes into use.
Conclusion
Computational imaging is a not-so-recent sub-field of the established field that is imaging. No magic happens in computational imaging: numerical apertures are still limited and low light levels challenging, but interdisciplinarity and hardware improvements allow for clever ideas, old and new, to bring forward new, sometimes game-changing, solutions. The global computational photography, part of imaging, market size was indeed valued at USD 18.3 billion in 2023 and is projected to grow at a CAGR of 13.0% from 2023 to 2028. The main players in this field are investing heavily in the research and development of computational imaging technologies and products to gain a competitive edge in the market.
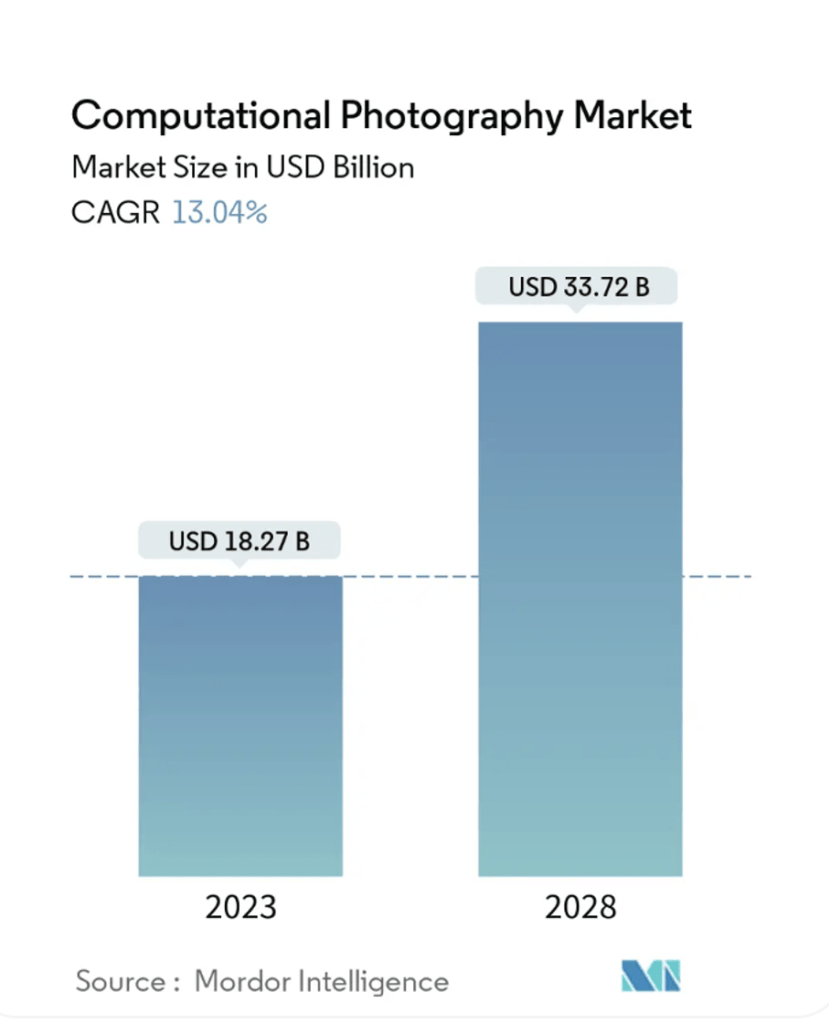
The computational imaging market has also witnessed the emergence of several new entrants and startups that have developed innovative products and solutions such as those highlighted in the use case part. These startups have raised significant funding and have achieved high valuations and revenues by offering cutting-edge computational imaging products and services [26, 27, 28].
Computational imaging innovations address markets where already-weathered solutions exist. Startups in this field should go much further than the prototypes and proofs-of- concepts. They should of course first prove that their innovations bring a tangible advantage in the field they address, but that they can also meet industry-relevant criteria (reliability, speed, repeatability, fabrication volumes, price, etc.). There is a long way between a lab experiment and a product, and the constraints of certain fields should be taken into account from inception: the distance they introduce can sometimes not be bridged [29].
Computational Imaging for MRI : Foqus
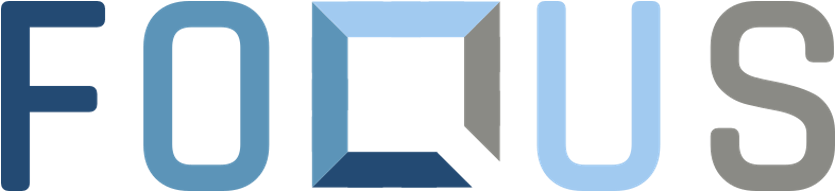
Foqus Technologies Inc. is building a software-based solution that leverages proprietary quantum technologies and artificial intelligence to enhance the quality and boost the sensitivity of magnetic resonance technology without requiring any sophisticated hardware upgrades.
Magnetic Resonance Imaging (MRI) is a cornerstone for non-invasive diagnostic medical imaging. Despite major hardware and pulse sequence design improvements [30,31], the acquisition of high-quality MRI still requires patients to remain motionless for at least 30 minutes.
Foqus has developed DeepFoqus, an innovative AI-powered MRI reconstruction software solution, leveraging advanced deep learning algorithms to accelerate MRI scanning process, while preserving image quality. With DeepFoqus, the scan time can be reduced up to 5 times. Below are the outcomes for the current version. In each test, the images present the same resolution although the one on the right was generated 4 times faster with DeepFoqus.
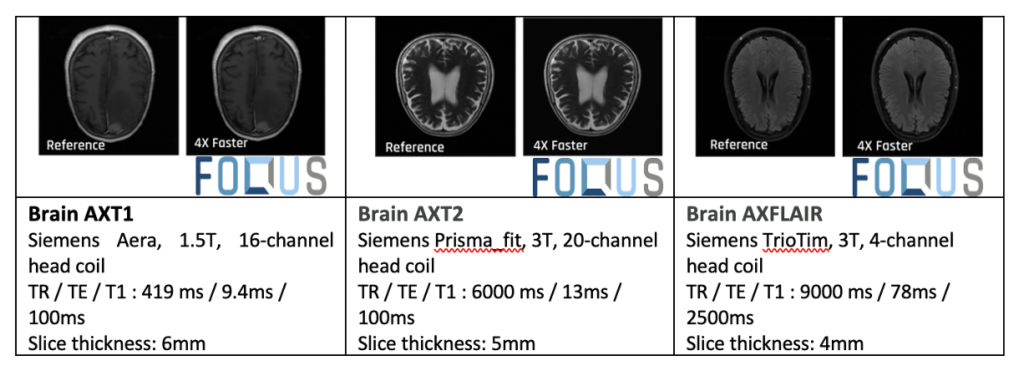
Foqus has also developed and patented a range of quantum sensing techniques that can improve the sensitivity and signal-to-noise ratio (SNR) of imaging biologically relevant molecules [32,33,34]. These technologies can be used to further speed up MRI scanning. Alternatively, it also enhances image quality. The increased sensitivity can broaden the scope of MRI applications.
For instance, the low sensitivity of magnetic resonance is the main obstacle for applications of MRI for metabolic imaging [35]. Foqus’s new technology enhances the sensitivity of MRI, allowing to image metabolites, with a total adressable market of MRI valued at US$ 7.9 Bn at the end of 2021 [36]. This expands the applications of MRI and opens new avenues to how we see and image the human body.
Acknowledgments
The authors thank Dr. Clémence Gentner, Dr. Hilton B. de Aguiar and Louis Delloye from Laboratoire Kastler Brossel (LKB) who helped to write this post and is grateful for the participation of Foqus’ team in its highlight.